How to build responsible AI practices into your organization
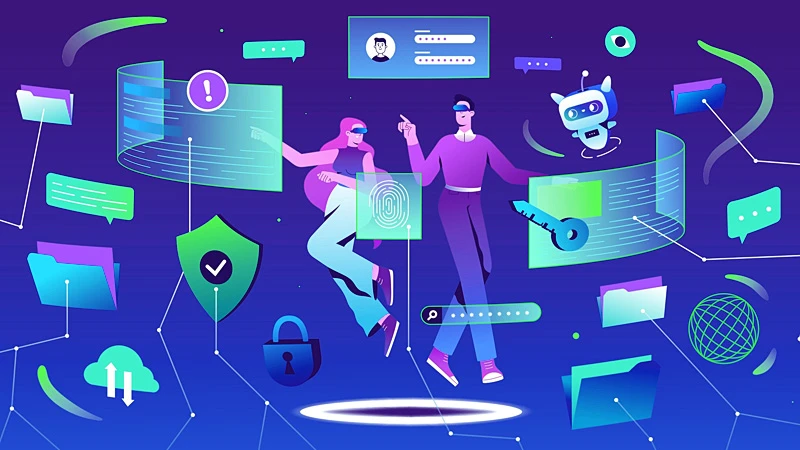
According to a 2024 McKinsey survey of over 100 organizations with more than $50 million in annual revenue, 63% of respondents have prioritized implementing generative AI (GenAI) into their businesses. However, 91% indicated that they did not feel well prepared to do so responsibly.
The benefits of aligning your GenAI strategy with responsible AI practices can't be overstated. Doing so helps businesses mitigate potential risks associated with the technology, employ GenAI in a way that corresponds with your corporate values and goals and helps establish your brand as a leader in the ethical use of GenAI applications. Most important, this alignment helps foster trust with your customers, which is paramount to benefitting from what generative AI has to offer.
To date, consumers have proved wary of trusting generative AI technology. A study of GenAI end users conducted by technology analyst firm Valoir showed that more than half (51%) were concerned that GenAI technology would violate their privacy. Other concerns uncovered by the Valoir study were that GenAI would: act on its own without human intervention (45%), use data unethically (40%), hallucinate (39%) and make biased recommendations (37%).
Further, with the introduction of new regulations, organizations that aren't doing enough to ensure the AI technology they develop and implement is accurate and fair could face financial consequences. For example, organizations that don't comply with the European Union Artificial Intelligence Act (EU AI Act) could face financial penalties of up to €35,000,000 or 7% of their total worldwide revenue for the preceding financial year, whichever is higher.
The stakes for developing and deploying AI systems that cause no harm are higher than ever before. As a result, brands must implement practices that adhere to the principles of responsible AI.
What is responsible AI?
Responsible AI is the practice of developing, implementing and using artificial intelligence in an ethical manner with the intention of it benefitting society. This entails aligning its use not only with laws and regulations, but also societal values. "The White House, the European Union, governments around the world — all are drafting new directives for responsible AI development," explained Steve Nemzer, TELUS Digital's director of AI growth and innovation, in the webinar, Building trust in generative AI.
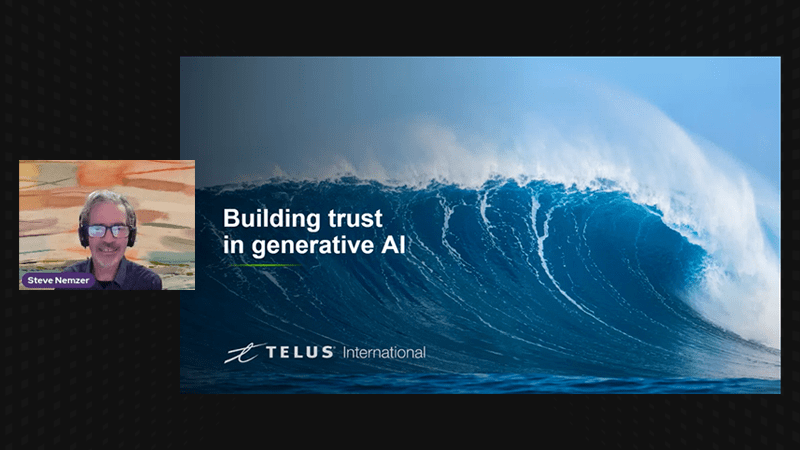
Building trust in generative AI
Brands are eager to reap the benefits of generative AI (GenAI) while limiting potential risks. Join Steve Nemzer, director of AI growth and innovation for TELUS Digital (formerly TELUS International), as he shares best practices for leveraging GenAI without compromising your organization’s goodwill.
As a result, practicing responsible AI necessitates a recognition of the technology's effect on stakeholders — from customers to employees to society at large. Central to this are some key principles, including the following.
Security and privacy
Adhering to robust data security and privacy practices is not only a critical component of responsible AI, it's also necessary for regulatory compliance. Examples include the General Data Protection Regulation (GDPR) in the European Union (EU), which upholds data privacy and security for EU citizens. In Canada, the Personal Information Protection and Electronic Documents Acts (PIPEDA) applies to private-sector organizations that, in the course of doing business, collect, use or disclose personal information. Such organizations must follow the 10 outlined principles of the Act to protect personal information.
Data security and privacy start with robust data governance practices. Businesses can leverage their existing data governance policies, incorporating additional practices specific to GenAI implementation. By doing so, they can build on proven internal practices and adapt them to changing regulatory requirements. For example, the tools you use, as stipulated by your governance practices, may need to be enhanced when preparing data for GenAI applications. This is because many non-traditional data management platforms, like vector databases (data stored as mathematical representations), are commonly used in building generative AI applications. It will likely be necessary to adapt best practices to meet the needs of this evolving technology.
Fairness
Data bias in GenAI models occurs when certain elements of a dataset are more heavily weighted as compared to others. This causes algorithms to perpetuate, or even amplify, existing social biases and inequalities. Consequently, these models don't accurately represent their use cases, and instead lead to skewed outcomes and low accuracy levels.
A recent UNESCO study looked at systematic prejudices in large language models (LLMs). Most LLMs are trained on astoundingly large datasets that reflect the biases of the population. Indeed, the study showed that one particular LLM was significantly more likely to associate gendered names with traditional roles. Specifically, female names were associated with home, family and children, while male names were associated with business, executive, salary and career.
Ensuring AI models produce fair decisions is imperative to combatting the pervasiveness and perpetuation of societal prejudices and inequities. It also serves your business, as a model that produces biased output is not one that is performing optimally for your organization.
Increased awareness: Transparency, explainability and accountability
As GenAI becomes more embedded in our daily lives, the demand for transparency continues to grow. This is unsurprising considering that transparency is critical to earning customer trust. "Vague information about plans around AI and generative AI can lead to worst-case scenario kinds of speculation," said Nemzer in the webinar. "Openly communicating about how generative AI will be used within a company right from the start will help build trust."
Consumers want to know when, and for what purpose, AI is being used. For example, a TELUS Digital survey showed that almost three-quarters (71%) of respondents agree it's important for companies to be transparent with consumers about how they are using GenAI. Additionally, a survey conducted by StoryStream, a marketing content platform service, showed that over half (58%) of respondents were more likely to trust brands that openly disclose the use of generative AI in their marketing.
Further, many governments advise the open disclosure of GenAI use. For example, in its Guide on the use of generative artificial intelligence within federal institutions, the Canadian government's recommended approach to using GenAI tools includes identifying GenAI-produced content, informing users when they are interacting with an AI tool and providing information on institutional policies, appropriate usage, training data and the model itself when deploying GenAI technology.
Transparency goes hand-in-hand with AI explainability, the goal of which is to enable humans to be able to understand and manage the output of AI and GenAI models. "The main idea behind explainable AI is that AI applications and uses should not be mysterious black boxes," said Nemzer. "When AI is being used, all parties should be informed. And the strengths and limitations of the AI model should be clear."
Both transparency and explainability are critical to building consumer trust in GenAI. Understanding how GenAI arrives at its conclusions provides an opportunity for humans to assess the validity of those outputs and helps them identify and resolve any potential inaccuracies and biases. For example, if GenAI tools are being used to support decision-making, the Canadian federal government recommends documenting these decisions and being able to provide explanations for them.
Accountability refers to humans within an organization taking responsibility for all AI outcomes, which includes any unintentional output. For example, generative AI hallucinations — a phenomenon where an LLM outputs fabricated or inaccurate responses — can significantly erode trust and credibility. They can also lead to financial losses due to customer dissatisfaction, product returns or legal liabilities. "With any technology, mistakes can happen," said Nemzer. "It could be wrong or fabricated information, a misstep on sensitive issues or just a poor overall experience."
Robust data governance practices again come into play. These will help to ensure the model is trained or fine-tuned using high-quality, diverse datasets to minimize inaccuracies and biases. Continual monitoring of GenAI output will allow businesses to rapidly respond to output deviations.
Having an understanding of some of the key principles of responsible AI is critical. Even more critical is implementing them into your businesses. While doing so takes time, resources and effort, the benefits in terms of risk mitigation are invaluable.
How to implement responsible AI
The implementation of responsible AI practices is critical to regulatory compliance, brand protection and fostering trust with customers. While the steps involved will be different for each business, there are some general best practices that should be considered across the board.
Align your leaders
Agreement from the leadership team is crucial when implementing a responsible AI framework, as this team is ultimately accountable for ensuring the business implements and adheres to these practices. Additionally, leaders are in charge of fostering a corporate culture that values responsible AI. They're also the ones to advocate for resources and ensure responsible AI practices are fundamental in decision-making.
Establish a responsible AI governance framework
The first step is to establish a governance team responsible for developing and enforcing responsible AI policies and best practices. Members of this team need to be assigned specific roles and responsibilities to oversee AI governance and compliance with responsible AI best practices. The team will need to take corporate values into account, as well as applicable laws and regulations.
Establishing your framework necessitates translating high-level principles into practical guidelines for responsible AI. For example, policies could include testing requirements prior to deploying GenAI technologies, conducting regular audits of GenAI applications, data security and privacy standards and processes for holding employees accountable.
Additionally, as global policymakers issue increasing guidance on the development and usage of GenAI, it's critical to keep track of the evolving regulatory environment and implement changes, as needed.
Put these principles into daily practice
It's one thing to develop a responsible AI governance framework, it's another to implement these principles into daily operations. Your organization's internal policies should not only include responsible AI best practices, but also instruct employees on how to embed these principles into their day-to-day functions.
Further, employees who may need to use GenAI should be provided with training regarding how these applications work, and when and how they should be used. Employees should also be educated about the potential occurrence of hallucinations and encouraged to think critically when assessing outputs.
Fostering an environment of open communication and learning can enhance awareness of responsible AI practices and empower employees to adhere to them.
Advance the performance, accuracy and safety of your generative AI model
Looking for a partner that understands and can deliver innovative offerings that prioritize responsible AI? With our deep AI experience, diverse workforce and cutting-edge technology for fine-tuning data tasks, we can help you build responsible AI.
Specifically, with our Fine-Tune Studio (FTS) platform, we are able to provide high-quality and diverse datasets, perform supervised fine-tuning to advance the human-like qualities of your GenAI application, reduce hallucinations via reinforcement learning from human feedback and implement guardrails through red teaming. Complementing FTS is Experts Engine, our sourcing platform that algorithmically matches tasks to be performed to the best qualified individuals from a diverse group of people.
With responsible AI practices at the forefront of what we do, we can help you improve your model's performance, adaptability and safety. Contact us to discuss your GenAI project.