Effectively applying human intelligence for evolving generative AI training
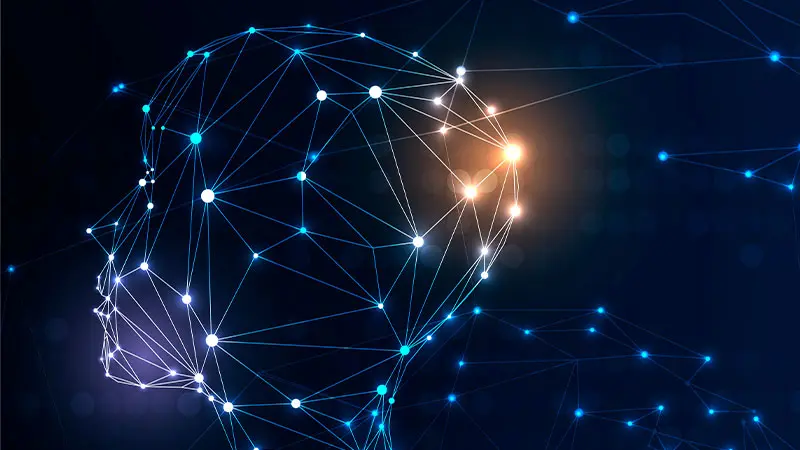
The development of artificial intelligence (AI) is progressing through key stages, fundamentally reshaping our approach to technology and problem solving. What began as basic chatbot interactions is now advancing toward fully autonomous decision-making systems to become truly agentic.
Looking ahead, we anticipate the emergence of innovative AI breakthroughs, which promise to accelerate advancements in material science, life sciences and physics. A prime example is AlphaFold, an AI model by DeepMind and Isomorphic Labs, which can predict a protein's 3D structure based on its amino acid sequence. The final evolution is organizational AI, envisioned to autonomously build and run entire companies.
The release of new reasoning models marks a significant milestone in this progression. These models are capable of solving complex, multimodal problems across coding, math and science. In fact, as Steve Nemzer, director of AI growth and innovation for TELUS Digital, outlines in the Are you ready for the era of Agentic AI? webinar, "Frontier models are solving 50% or more of the reasoning problems that would challenge a Ph.D.-level candidate in STEM fields.”
Having been in the data collection and labeling industry for over two decades, we recognize that high-quality data curated by specialized experts is a driving force behind these improvements. In this article, we’ll explore how expert-driven training data is transforming AI models, enhancing their reasoning capabilities and paving the way for agentic AI.
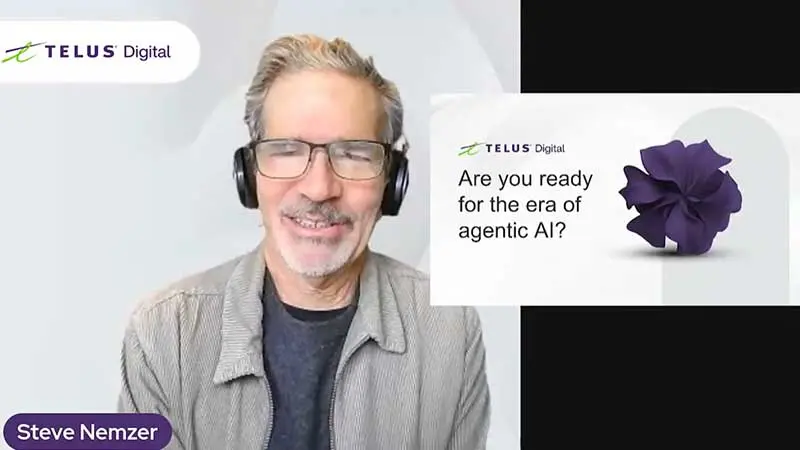
Are you ready for the era of agentic AI?
Join Steve Nemzer, director of AI growth and innovation for TELUS Digital, as he unveils the transformative world of agentic artificial intelligence (AI). Learn what makes AI “agentic”, various use cases and implementation best practices to benefit your organization.
How human expert oversight shapes AI models
As AI development evolves, the focus is shifting away from large-scale, mass data labeling and toward smaller-scale annotation done by specialized experts in fields like science, technology, engineering, mathematics (STEM) and coding to enhance model reasoning and accuracy. The focus is no longer on sheer volume, such as relying on thousands of generalist annotators. Instead, developers are leveraging the insights of highly-skilled experts to identify model weaknesses, provide precise, high-quality feedback and test models to the edge of their capabilities. These experts don’t simply label — they create training data and touchpoints that guide the model towards better reasoning, refining and verification.
This shift underscores a fundamental truth that AI is not an autonomous force but a distilled, statistical representation of collective human judgment and intelligence. At its core, AI models, particularly large language models (LLMs), learn by imitating patterns in data that human annotators have curated, annotated or structured. These experts, with domain-specific knowledge, imbue AI systems with nuanced understanding and practical utility. While advanced techniques like reinforcement learning further refine these models, their foundational knowledge originates from expert input. Ultimately, as AI continues to evolve, the benchmark for intelligence remains humanity itself — making the creation of frontier data a fascinating fusion of human expertise and advanced algorithms.
Teaching AI to self-correct
Beyond structured reasoning, a major challenge for today’s LLMs is intrinsic self-correction: the ability to recognize and fix their own mistakes without external human input. Research, like Google DeepMind’s Self-Correction via Reinforcement Learning (SCoRe) approach, has shown that multi-turn reinforcement learning — where models generate an initial response and iteratively revise it using reward shaping — can significantly improve accuracy. Similarly, advanced prompting strategies, such as “self-refine,” allow models to critique and enhance their answers through multiple iterations before reaching a final response.
Building on these insights, here’s an example of how a bespoke expert-driven approach can be applied to train an AI model:
- Complex prompts on advanced topics created by domain experts can be used as model inputs.
- Relevant context and guidelines for the response can be set by the expert. Using this context and the input prompt, the model generates an initial response.
- The model-generated responses can then undergo multiple rounds of iterative review involving expert annotators, reviewers and quality-control teams.
- The annotators can analyze the reasoning steps in the response, suggest corrective measures as “annotations” to reinforce the model’s reasoning behind each action.
- The LLM can then regenerate responses using these corrective measures and this process can iteratively continue until a satisfactory response is provided.
By enforcing structured step-by-step validation, targeted feedback and multiple correction loops, developers can enable the model to improve its reasoning, refine its problem-solving approach and enhance its ability to self-correct errors efficiently.
Enhancing AI reasoning with structured data and expert sourcing
Advancing AI reasoning and problem solving depends on enabling models to explore complete action sequences through chain-of-thought (CoT) and tree-of-thought (ToT) training. By systematically generating CoT and ToT templates, developers have significantly enhanced large language models’ ability to reason step by step, allowing them to navigate complex queries and produce verifiable responses.
True human-like problem solving draws on adaptive strategies that combine diverse expertise, integrate interdisciplinary approaches and leverage a broad knowledge base. This dynamic process involves:
- Drawing from a variety of knowledge bases and techniques.
- Experimenting with different methods to see what works best.
- Recognizing when an approach isn’t working and being willing to change course.
- Identifying when a method is leading to progress and building on it.
- Knowing when we’ve reached a valid answer and confidently stopping there.
Recognizing the need to capture such nuanced human reasoning at scale, at TELUS Digital, we have introduced two innovative solutions:
- We developed an off-the-shelf STEM dataset that features over 80,000 comprehensive multimodal prompt-response pairs covering physics, mathematics, chemistry, biology, coding and various reasoning tasks. Designed using an incremental task-training format that spans difficulty levels from general to expert-level challenges, the dataset enables models to progressively learn and refine their STEM and reasoning capabilities — from generating responses to making complex, step-by-step decisions.
- Our specialized talent-matching platform, Experts Engine, streamlines the sourcing and qualification of human experts for fine-tuning and model evaluation. By programmatically matching the right talent from thousands of AI community contributor profiles to generative AI training projects, our platform ensures access to top-tier human intelligence. The process begins with automated talent extraction that identifies key parameters for informed resource planning, followed by sourcing specialists using a proprietary explorer dashboard to locate the best-fit experts. This continuously updated database, enriched by insights from multiple projects, guarantees ongoing access to highly-qualified contributors. Rigorous qualification processes and fraud-prevention measures further ensure that only verified experts contribute to training AI models.
Together, these solutions empower model builders to access and deploy specialized AI expertise with unprecedented efficiency, transforming the challenge of sourcing top-tier human intelligence into a streamlined, scalable process. At TELUS Digital, our comprehensive ecosystem delivers high-quality AI training data solutions and an advanced Experts Engine that connects you with rigorously vetted domain-specific specialists. This unique combination enables faster model refinement, enhanced reasoning capabilities and improved self-correction performance while reducing training time and boosting overall accuracy. By partnering with us, you gain access to state-of-the-art tools and a continuously updated pool of expert talent, ensuring your AI models remain at the cutting edge of agentic, self-improving technology.
Connect with us to explore how TELUS Digital’s offerings can drive breakthrough innovations in reasoning, coding, problem solving and beyond.